The Unfulfilled Promise Of Reinforcement Learning In AI Model Enhancement
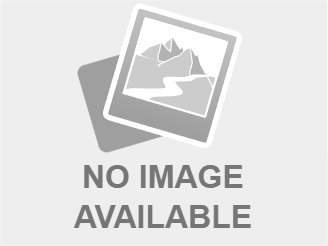
Welcome to your ultimate source for breaking news, trending updates, and in-depth stories from around the world. Whether it's politics, technology, entertainment, sports, or lifestyle, we bring you real-time updates that keep you informed and ahead of the curve.
Our team works tirelessly to ensure you never miss a moment. From the latest developments in global events to the most talked-about topics on social media, our news platform is designed to deliver accurate and timely information, all in one place.
Stay in the know and join thousands of readers who trust us for reliable, up-to-date content. Explore our expertly curated articles and dive deeper into the stories that matter to you. Visit NewsOneSMADCSTDO now and be part of the conversation. Don't miss out on the headlines that shape our world!
Table of Contents
The Unfulfilled Promise of Reinforcement Learning in AI Model Enhancement: A Reality Check
Reinforcement learning (RL), a powerful machine learning technique, has been touted as the key to unlocking the next generation of AI. The promise? To significantly enhance the performance and adaptability of existing AI models, pushing them beyond their current limitations. However, the reality is proving more complex, revealing a gap between the hype and the tangible results. This article delves into the challenges hindering the widespread adoption of RL for AI model enhancement and explores potential pathways forward.
Reinforcement learning operates on a feedback loop: an agent interacts with an environment, receives rewards for desirable actions, and learns an optimal policy to maximize its cumulative reward. This seemingly straightforward approach holds immense potential for improving AI models across diverse applications, from optimizing robotic control systems to personalizing user experiences. However, several hurdles are significantly slowing its progress.
The Challenges Facing RL in AI Model Enhancement
1. Sample Inefficiency: RL algorithms often require a vast number of interactions with the environment to learn effectively. This translates to substantial computational resources and time, making it impractical for many real-world applications. Training sophisticated AI models using RL can be prohibitively expensive, especially when dealing with complex, high-dimensional environments.
2. Reward Function Engineering: Defining an appropriate reward function is crucial for successful RL. A poorly designed reward function can lead the agent to learn suboptimal or even unintended behaviors. Crafting effective reward functions is a highly specialized skill and requires deep understanding of both the AI model and the task at hand. This remains a significant bottleneck in widespread RL implementation.
3. Exploration-Exploitation Dilemma: RL agents must balance exploration (trying new actions to discover potentially better strategies) and exploitation (using the currently best-known strategy). Striking the right balance is critical for efficient learning, but often proves difficult to achieve in practice. An agent that explores too much might miss out on optimal solutions, while one that exploits too much might get stuck in local optima.
4. Generalization and Transfer Learning: RL agents often struggle to generalize their learned policies to new, unseen situations. This limits their applicability and necessitates retraining for every new scenario. Effective transfer learning techniques, allowing knowledge gained in one environment to be applied to another, remain an active area of research.
5. Safety and Stability: In critical applications such as autonomous driving or medical diagnosis, the safety and stability of RL agents are paramount. Ensuring that an RL agent will not exhibit unexpected or dangerous behavior is a complex challenge, requiring robust safety mechanisms and extensive testing.
The Path Forward: Bridging the Gap
Despite these challenges, the potential of RL for AI model enhancement remains significant. Several promising avenues are being explored:
- Improved algorithms: Researchers are actively developing more sample-efficient and robust RL algorithms. Techniques like off-policy learning and model-based RL aim to address the limitations of traditional approaches.
- Meta-learning for RL: Meta-learning aims to learn how to learn, enabling RL agents to adapt more quickly to new environments and tasks. This could significantly improve generalization capabilities.
- Sim-to-real transfer: Utilizing simulations to train RL agents before deploying them in real-world environments can reduce the need for extensive real-world data and improve safety.
- Human-in-the-loop RL: Incorporating human feedback into the RL process can improve the design of reward functions and guide the agent towards safer and more desirable behaviors.
In conclusion, while the initial promise of revolutionizing AI model enhancement through reinforcement learning hasn't been fully realized, significant progress is being made. Addressing the challenges outlined above, through both algorithmic innovations and a deeper understanding of RL's limitations, is crucial to unlocking the true potential of this powerful technique and realizing its transformative impact on the field of artificial intelligence. The journey continues, but the destination remains a compelling goal.
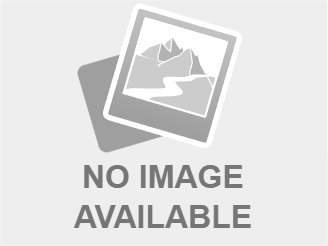
Thank you for visiting our website, your trusted source for the latest updates and in-depth coverage on The Unfulfilled Promise Of Reinforcement Learning In AI Model Enhancement. We're committed to keeping you informed with timely and accurate information to meet your curiosity and needs.
If you have any questions, suggestions, or feedback, we'd love to hear from you. Your insights are valuable to us and help us improve to serve you better. Feel free to reach out through our contact page.
Don't forget to bookmark our website and check back regularly for the latest headlines and trending topics. See you next time, and thank you for being part of our growing community!
Featured Posts
-
Kepa Arrizabalaga To Bournemouth Transfer Snag Emerges
May 02, 2025 -
Ge 2025 Key Rules To Remember During The Election Cooling Off Period
May 02, 2025 -
Exclusive Lewis Pullmans Sentry Costume Revealed In Thunderbolts Figurine
May 02, 2025 -
Real Betis Vs Fiorentina Probable Starting Lineups For Conference League Semi Final
May 02, 2025 -
Chelseas True Mettle Djurgarden And Liverpool Tests Await
May 02, 2025
Latest Posts
-
The Walking Dead Dead City Season 2 Episodes 1 6 Review A Look At The New Season
May 03, 2025 -
Ge 2025 Dpm Gans Punggol Shift A Strategic Decision Or Sign Of Diminished Influence Pritams Analysis
May 03, 2025 -
The Movie Title Sequel A Disappointing Return
May 03, 2025 -
May Showers Likely Met Service Forecasts Thundery Downpours
May 03, 2025 -
Sui Token Price Prediction Experts See Potential For 4
May 03, 2025