Re-evaluating Reinforcement Learning's Impact On AI Model Performance
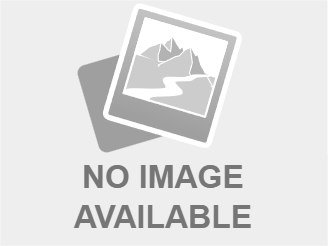
Welcome to your ultimate source for breaking news, trending updates, and in-depth stories from around the world. Whether it's politics, technology, entertainment, sports, or lifestyle, we bring you real-time updates that keep you informed and ahead of the curve.
Our team works tirelessly to ensure you never miss a moment. From the latest developments in global events to the most talked-about topics on social media, our news platform is designed to deliver accurate and timely information, all in one place.
Stay in the know and join thousands of readers who trust us for reliable, up-to-date content. Explore our expertly curated articles and dive deeper into the stories that matter to you. Visit NewsOneSMADCSTDO now and be part of the conversation. Don't miss out on the headlines that shape our world!
Table of Contents
Re-evaluating Reinforcement Learning's Impact on AI Model Performance: A Critical Look at Current Trends
Reinforcement learning (RL), a powerful technique enabling AI agents to learn through trial and error, has garnered significant attention in recent years. While promising breakthroughs have been achieved, a critical re-evaluation of its true impact on overall AI model performance is necessary. This article delves into the current landscape, exploring both the successes and limitations of RL in boosting AI capabilities.
The Hype vs. the Reality: RL's Promising Potential and Present Challenges
The allure of RL lies in its ability to train AI agents to solve complex problems, often surpassing human capabilities in specific tasks. From mastering games like Go and chess to optimizing robotic control systems and resource allocation, RL has demonstrably improved performance in numerous domains. However, the path to achieving these successes is often fraught with challenges.
-
Data Dependency: RL algorithms heavily rely on vast amounts of data for effective training. Acquiring and processing this data can be expensive, time-consuming, and sometimes ethically problematic. The sheer volume needed for optimal performance presents a significant hurdle for many applications.
-
Sample Inefficiency: RL agents often require a considerable number of training iterations to converge on optimal solutions. This sample inefficiency can significantly slow down the development process and make it computationally expensive, especially for complex tasks.
-
Reward Shaping Challenges: Defining appropriate reward functions is crucial for RL’s success. Poorly designed reward functions can lead to unintended consequences, where the agent finds loopholes to maximize rewards without achieving the desired behavior. This "reward hacking" phenomenon highlights the critical need for careful reward function engineering.
-
Generalization Issues: An AI agent trained using RL in a specific environment might struggle to adapt to slightly different conditions. This lack of generalization poses limitations on the broad applicability of RL-trained models.
H2: Beyond Game Playing: Exploring Real-World Applications and Limitations
While RL has achieved impressive feats in the gaming world, its real-world impact remains a subject of ongoing research. Applications in robotics, autonomous driving, and personalized medicine show promise, but also highlight some key limitations:
-
Robotics: RL is used to improve robotic dexterity and control, enabling robots to perform complex manipulation tasks. However, safety and reliability remain crucial concerns, requiring robust solutions to handle unexpected situations.
-
Autonomous Driving: RL offers the potential for creating safer and more efficient autonomous driving systems. However, the need for extensive real-world testing and the complexities of handling unpredictable road conditions pose significant challenges.
-
Personalized Medicine: RL can personalize treatment plans based on individual patient data, potentially improving treatment outcomes. However, the need for robust data privacy and ethical considerations are paramount.
H2: Future Directions: Bridging the Gap Between Promise and Practicality
To fully unlock RL's potential, researchers are actively pursuing several promising directions:
-
Improved Algorithms: Developing more sample-efficient algorithms will significantly reduce training time and computational costs.
-
Transfer Learning: Enabling RL agents to transfer knowledge learned in one environment to another will enhance their adaptability and generalization capabilities.
-
Safe RL: Developing methods to ensure safe and reliable RL agents is crucial for their deployment in real-world applications. This includes robust handling of unexpected events and error recovery mechanisms.
-
Explainable RL (XRL): Making RL algorithms more transparent and interpretable will foster trust and facilitate debugging.
Conclusion:
Reinforcement learning offers significant potential for advancing AI capabilities. However, its practical impact is currently limited by challenges related to data dependency, sample inefficiency, reward shaping, and generalization. Addressing these limitations through ongoing research and development is crucial for realizing the full promise of RL and unlocking its transformative power across various domains. Continued focus on algorithm improvement, transfer learning, safety mechanisms, and explainability will be key to achieving truly impactful AI systems.
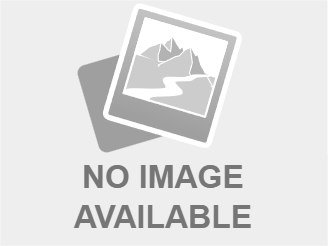
Thank you for visiting our website, your trusted source for the latest updates and in-depth coverage on Re-evaluating Reinforcement Learning's Impact On AI Model Performance. We're committed to keeping you informed with timely and accurate information to meet your curiosity and needs.
If you have any questions, suggestions, or feedback, we'd love to hear from you. Your insights are valuable to us and help us improve to serve you better. Feel free to reach out through our contact page.
Don't forget to bookmark our website and check back regularly for the latest headlines and trending topics. See you next time, and thank you for being part of our growing community!
Featured Posts
-
The Security Implications Of Using Ai Models With Key Permissions In Web3
May 02, 2025 -
Uefa Conference League Djurgarden Vs Chelsea Complete Head To Head Analysis
May 02, 2025 -
Gosens E La Fiorentina Sfiorano Il Pari Contro Il Betis 2 1 Il Risultato Finale
May 02, 2025 -
Europa League Semi Final Can Tottenham Overcome Bodo Glimt In The First Leg
May 02, 2025 -
Amazon Invests Heavily In Satellite Network To Reduce Reliance On Elon Musks Starlink
May 02, 2025
Latest Posts
-
Trumps Presidency A Key National Security Advisor Departure And Un Appointment
May 02, 2025 -
Court Orders Haliburtons Father To Avoid Pacers Games And Arena
May 02, 2025 -
Free Software Update Boosts Amazons Top Rated Tv
May 02, 2025 -
Pga Club Professional Championship Collet Takes Home The Title 20 Players Qualify For Major
May 02, 2025 -
Brave Festival Attendee Stops Suspects Escape At Lapu Lapu Event
May 02, 2025