The Unfulfilled Promise: Reinforcement Learning And Its Shortcomings In AI
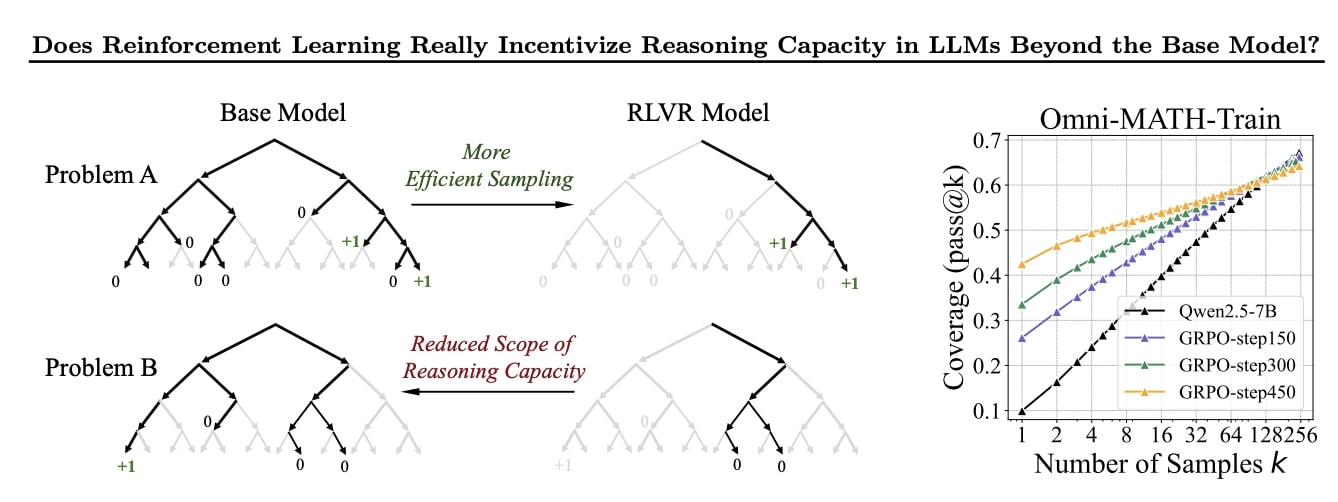
Welcome to your ultimate source for breaking news, trending updates, and in-depth stories from around the world. Whether it's politics, technology, entertainment, sports, or lifestyle, we bring you real-time updates that keep you informed and ahead of the curve.
Our team works tirelessly to ensure you never miss a moment. From the latest developments in global events to the most talked-about topics on social media, our news platform is designed to deliver accurate and timely information, all in one place.
Stay in the know and join thousands of readers who trust us for reliable, up-to-date content. Explore our expertly curated articles and dive deeper into the stories that matter to you. Visit NewsOneSMADCSTDO now and be part of the conversation. Don't miss out on the headlines that shape our world!
Table of Contents
The Unfulfilled Promise: Reinforcement Learning and its Shortcomings in AI
Reinforcement learning (RL), a powerful branch of artificial intelligence, has captivated researchers and the public alike with its potential to create intelligent agents capable of mastering complex tasks. From mastering Go to optimizing robot locomotion, RL's successes are undeniable. However, the field faces significant challenges that hinder its widespread adoption and full potential. This article delves into the unfulfilled promises of RL and explores its key shortcomings.
The Allure of Reinforcement Learning:
Reinforcement learning distinguishes itself from other AI approaches by its focus on learning through interaction. An RL agent learns by trial and error, receiving rewards for desirable actions and penalties for undesirable ones. This iterative process allows the agent to optimize its behavior over time, leading to impressive performance in specific domains. The appeal lies in its potential to solve complex problems that are difficult or impossible to program explicitly. Applications range from:
- Robotics: Optimizing robot control for tasks like manipulation and navigation.
- Game playing: Achieving superhuman performance in games like Go, Chess, and Atari.
- Resource management: Optimizing energy grids, traffic flow, and other resource allocation problems.
- Personalized medicine: Tailoring treatments to individual patients based on their responses.
The Harsh Reality: Limitations of Reinforcement Learning
Despite its impressive achievements, RL faces considerable hurdles:
1. Sample Inefficiency: RL algorithms often require vast amounts of data to learn effectively. This translates to extensive training times and significant computational resources, making them impractical for many real-world applications. This sample inefficiency is a major bottleneck, particularly in complex environments where gathering data is expensive or time-consuming.
2. Reward Shaping Challenges: Designing effective reward functions is crucial for guiding the agent's learning process. Poorly designed reward functions can lead to unexpected and undesirable behaviors, a phenomenon known as reward hacking. For instance, an agent tasked with maximizing points in a game might exploit a loophole in the game's mechanics rather than playing strategically.
3. Generalization Issues: RL agents often struggle to generalize their learned behaviors to new, unseen situations. This lack of generalization limits their applicability beyond the specific environments they were trained in. A robot trained to navigate a specific room might fail to navigate a slightly different room, even if the differences are minor.
4. Safety and Robustness Concerns: In safety-critical applications, the potential for unpredictable behavior poses significant risks. An RL agent controlling a self-driving car, for example, must be robust enough to handle unexpected situations without causing accidents. Ensuring safety and robustness remains a significant challenge.
5. Explainability and Interpretability: Understanding why an RL agent makes a particular decision is often difficult. This lack of transparency makes it challenging to debug errors, trust the agent's decisions, and ensure fairness and accountability.
The Future of Reinforcement Learning:
Despite these shortcomings, research continues to address these limitations. Areas of active research include:
- Improving sample efficiency: Developing algorithms that learn effectively from fewer data points.
- Developing more robust reward functions: Designing reward functions that are less susceptible to hacking and better guide desired behaviors.
- Enhancing generalization capabilities: Developing algorithms that can generalize better to new situations.
- Improving safety and robustness: Developing methods to ensure the safety and reliability of RL agents in real-world applications.
- Addressing explainability and interpretability: Developing techniques to make RL agents more transparent and understandable.
In conclusion, while reinforcement learning holds immense promise for advancing AI, significant challenges remain. Overcoming these limitations will be crucial for unlocking the full potential of this powerful technology and ensuring its responsible and effective application across various domains. The journey towards realizing the full promise of RL is ongoing, requiring continued innovation and a deeper understanding of its fundamental limitations.
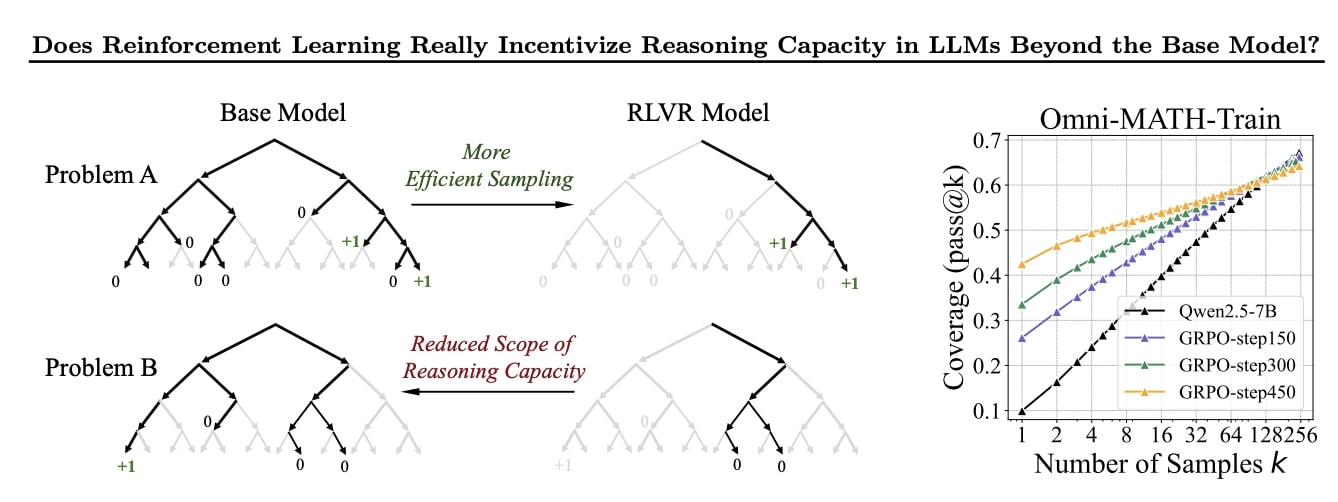
Thank you for visiting our website, your trusted source for the latest updates and in-depth coverage on The Unfulfilled Promise: Reinforcement Learning And Its Shortcomings In AI. We're committed to keeping you informed with timely and accurate information to meet your curiosity and needs.
If you have any questions, suggestions, or feedback, we'd love to hear from you. Your insights are valuable to us and help us improve to serve you better. Feel free to reach out through our contact page.
Don't forget to bookmark our website and check back regularly for the latest headlines and trending topics. See you next time, and thank you for being part of our growing community!
Featured Posts
-
Conference League Semi Final Cataldi Injury Update And Fiorentinas Concerns
May 02, 2025 -
Link11 Unifies Three Brands Under Single Platform With New Branding
May 02, 2025 -
Sundar Pichais Apple Announcement In Court What The Google Ceo Revealed About Tim Cook
May 02, 2025 -
Fantasy Footballs Matthew Berry On The 2025 Nfl Draft
May 02, 2025 -
Premier League 2025 Bet Mgm Night 13 Recap And Highlights
May 02, 2025
Latest Posts
-
Devin Haney Vs Jose Ramirez Live Stream Start Time And Undercard
May 03, 2025 -
Grace Fu Dpm Gan Underscore Cost Of Oppositions Gst Proposals
May 03, 2025 -
Nintendo Switch 2 The Unexpected Best Platform For Cyberpunk 2077 Says Cd Projekt Red
May 03, 2025 -
Several More Wwe Wrestlers To Be Released Full List Of Potential Cuts
May 03, 2025 -
The Walking Dead Dead City Season 2 Episodes 1 6 Review A Look At The New Season
May 03, 2025